Geosocial Segmentation Whitepaper
Location intelligence is vital to the success of any business that sells physical products or services. They rely on accurate location data to optimize distribution, plan site selection, and extract market insights. This data comes at a premium. The U.S. census, a primary source of location intelligence across industries, cost the government over $13B. Companies spend millions to conduct surveys of communities just to aggregate a useful sample. Recently, companies have begun using mobile location data to understand human movement, despite the high price and privacy concerns.
Consider if there was a source of data, composed of billions of data points across the entire U.S. and much of the world, where people were publicly and knowingly sharing the behaviors, experiences, and personalities that take place in their neighborhoods. This dataset exists and has existed since at least 2009. It is social media data that has a location associated with it, otherwise known as Geosocial data. It is contributed to organically, unlike the census or surveys, removing bias. It is open and public data, that anyone could access right now for free, unlike costly and exclusive mobile location data.
The problem companies face when using this data is that is a very large source of unstructured text data. To make adequate use of the data companies need to be able to extract insight from content and organize this information into a format that can be mapped or used in analytics. This is the problem Spatial.ai set out to solve.
Testing
Spatial.ai works with some of the largest companies in the U.S. to help them understand location performance. Through this work and authorized usage of their performance indicators (in most cases this is revenue), we were able to create a benchmark to test the effectiveness of any methods attempted. Results of all tests could be compared to location-based business performance to see how much it could account for real-world outcomes. Additional desired criteria were to minimize human bias, to use as much of the data as possible, and to tie the output to actionable insights.
Approaches
Approach 1 - Human defined segments
Our team consists of ethnographers and data scientists, so as a first approach, we used human researchers to identify posts and terms used on social media that could signify types of behavior. This resulted in a handful of categories of social activity that when tested against business performance, demonstrated the ability to predict outcomes with moderate success. The two primary problems identified with this approach were that it contained human bias (humans were deciding what information was important initially) and that it did not make use of any data that was not chosen by the researcher, limiting how much information was contained in the end product.
Approach 2 - Supervised learning
The second approach used supervised machine learning to extend the efforts of the research team. Researchers categorized posts and topics to train a machine learning model to differentiate themes between posts. Over time, the model began to understand more complete behaviors and relationships between topics. For example, it could accurately categorize “gears” as a topic related to bicycling even though the researcher never identified a social media post that included the term. When tested against business performance, it had a 300% improvement over the previous method. This was considered a strong candidate for the permanent solution. However, this approach still contains human biases. People are still deciding which data matters the most. And a human can only categorize a small sample of the data, so there were insights that we were still missing.
Approach 3 - Unsupervised learning
The final approach was unsupervised learning. Using this method, we were able to use all of the dimensions of the data, including phrases, terms, time, and proximity. After many iterations of this approach, the team produced a dataset of over 70 social media “segments” that were then passed to the research team. The research team was easily able to identify the themes or behaviors that the machine learning process identified from human conversation. Now, instead of trying to figure out which data to use, the researchers simply had to help interpret and communicate the data the machine had organized organically. This satisfied our requirement for reducing human bias. Now, the ultimate test was to compare the results for predicting business outcomes. Not only did it outperform the previous best method by 30%, but for some clients, it proved more insightful as a single source of information than data from the census.
Conclusion
Using the results of these tests, Geosocial data has been organized with unsupervised learning and is now available in 70+ segments of activity that can be provided as percentiles for any geographic unit across the entire U.S. and Canada. Clients are using this data to successfully map behaviors in their markets and predict business performance.
To explore these segments visit our taxonomy.
To learn more about Geosocial data, visit our Essential Guide to Geosocial Data.
What you should do now
Whenever you're ready, here are 3 ways Spatial.ai can help:
- Schedule your free PersonaLive demo. Discover how to identify, analyze, and target your most valuable customers in under 60 minutes with the PersonaLive platform. During your demo, we'll review your existing customer data and suggest actionable segmentation strategies to help you reach your marketing goals.
- If you'd like more segmentation strategies, go to our resources section, where you can access webinars, downloadable guides, and product tutorials.
- If you know another marketer who’d enjoy reading this post, share it with them via Linkedin, X, or Facebook.
Get retail marketing tips
We email every monday with smart growth strategy ideas. Almost no promotion. Just value.
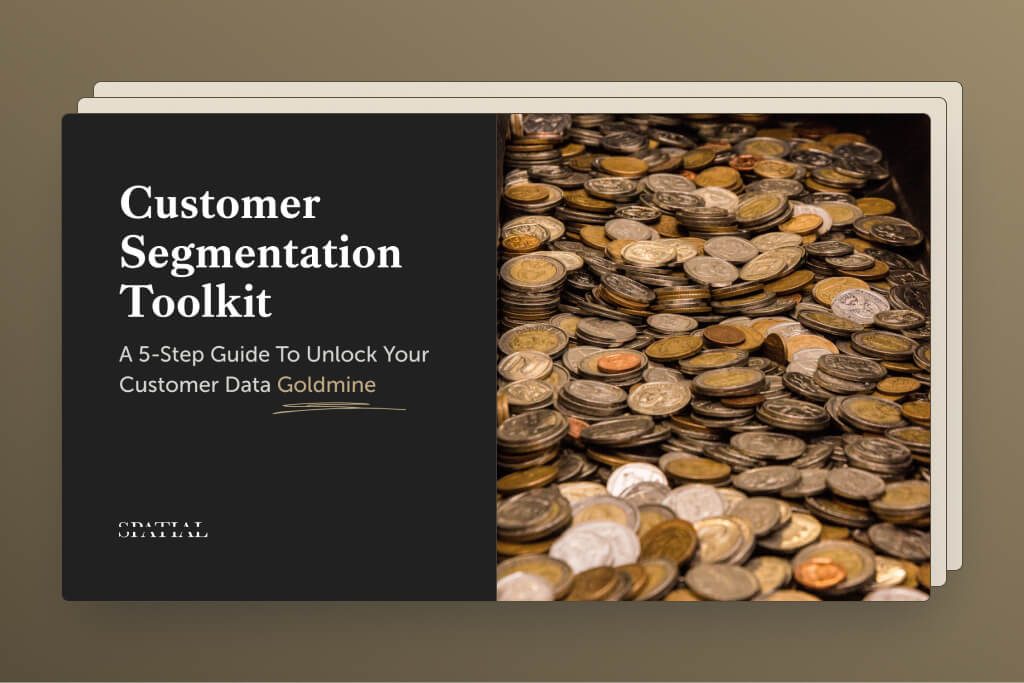
.avif)
%20(1).jpg)
