How AI Is Reshaping Retail Location Strategy, Pt 1
Retail real estate is at a turning point. In 2024, store closures and openings both hit record highs. With another 15,000 closures projected in 2025, every site decision matters more than ever.
Retailers are adapting by integrating relevant data and AI into their location strategy to simulate strategies, de-risk expansion, and outmaneuver competitors.
In this series, we’ll break down how AI is transforming each stage of the site expansion process:
- Building and ranking store databases
- Defining trade areas
- Analyzing customers
- Evaluating competitors
- Forecasting sales
- Prioritizing markets
Each post will explore the evolution from traditional methods to modern AI-driven approaches—covering the foundational fundamentals, new data sources, and how AI brings it all together.
Before we begin, let’s look at how we got here and the three ways AI can help.
{{schedule-demo}}
The Three Eras Of Location Research
Just as retail has evolved dramatically over the past two decades, so too has the process of retail real estate research. The journey can be understood in three distinct eras:

1950–2000: The Fundamentals Era
This era laid the groundwork for modern location strategy. Despite the surge in data and computing power since, the core principles remain the same. During this foundational period, the industry developed its standard toolkit, including:
- Trade area calculations
- Store database development
- Census-based demographic analysis
- Surveys and intercept methodology
- Regression modeling and sales forecasting
- Cannibalization and competitive impact modeling
- Geodemography and customer segmentation
These fundamentals still serve as the scaffolding for site selection today.
2000–2020: The Data Proliferation Era
The rise of digitization, and the ability to tag digital behavior to physical locations, ushered in a flood of new data sources. Location research evolved from “educated guesswork” to “data-backed modeling,” drawing on real-world behavioral inputs. Key data types introduced during this time include:
- Mobile foot traffic data
- Social media signals
- Listings and property data
- Loyalty data
- Purchase data
- Point-of-interest (POI) datasets
2020–Future: The AI Augmentation Era
While AI has been in development for decades, the breakthrough moment came with the release of tools like ChatGPT and the widespread adoption of large language models. The fundamentals haven’t changed—but our ability to analyze, simulate, and synthesize information has accelerated dramatically.
AI brings scale, speed, and stamina—handling complex analyses that would exhaust even the most seasoned analysts. Emerging use cases include:
- High-dimensional multivariate analysis
- Data cleaning and classification
- Scenario simulation at scale
- Real-time customer segmentation
- Variable interpretation and insight generation
In short:
- Era One gave us the fundamentals.
- Era Two gave us richer data.
- Era Three gives us the power to apply both—faster, smarter, and at scale.
Three Ways To Use AI In Location Research
Ultimately, AI fills three critical gaps in location research—areas where human effort and traditional tools alone aren’t enough.
1. Clean & Label
AI doesn’t get tired. That makes it especially good at the repetitive, time-consuming tasks that are essential to location strategy—but often slow teams down. While not always 100% accurate, AI delivers consistent, scalable results that save time and unlock new ways of thinking.
Clean data is the foundation of strong location analysis. AI can speed up this mundane process and save teams hundreds of hours of grunt work.
Here’s some examples of how we use it at Spatial.ai:
- Formatting: Upload a scraped list of competitor addresses to ChatGPT and have it reformat the columns to match your internal store database.
- Categorization: Provide a list of retailers or social media accounts, define your desired categories, and let ChatGPT sort them accordingly.
- Labeling: Share a screenshot of a retail property and ask AI to count the number of parking spots
2. Scale & Simulate
AI gives us the ability to up the scale, speed and accuracy of forecasting models. We can predict sales at more points (scale). We have the processing power and necessary algorithms to run the simulation faster (speed). And we can take more variables into account and look at the relationships between variables to improve forecasts (accuracy). Here are three examples.
- Simulation: Simulate forecasts across millions of seed points on a map.
- Interaction Effects: Test millions of variable combinations to forecast sales.
- Speed: Historically it could have taken ages to run this, now it could take minutes.
None of the above necessarily require the use of LLMs. But leverage their predecessors: machine learning algorithms and compute power.
3. Storytell & Hypothesize
What’s surprised us most is how AI can help connect the dots, especially when it comes to uncovering the why behind unexpected trends or supporting location storytelling.
While it’s not always accurate, it offers a range of possibilities that sharpen human intuition and often lead to the right insight.
- Variable explanation & hypothesis building: Say you find a non-intuitive variable is a major driver of sales. Before dismissing it, ask ChatGPT (with the right context) to generate 10 reasons it could be impacting the forecast.
- Visualization: Upload an image of an empty grocery anchor and prompt ChatGPT to visualize the space with a Trader Joe’s and an OrangeTheory. This is much better than a Google streetview screenshot.
- Summarization: AI is great at condensing survey responses or interview transcripts into key themes.
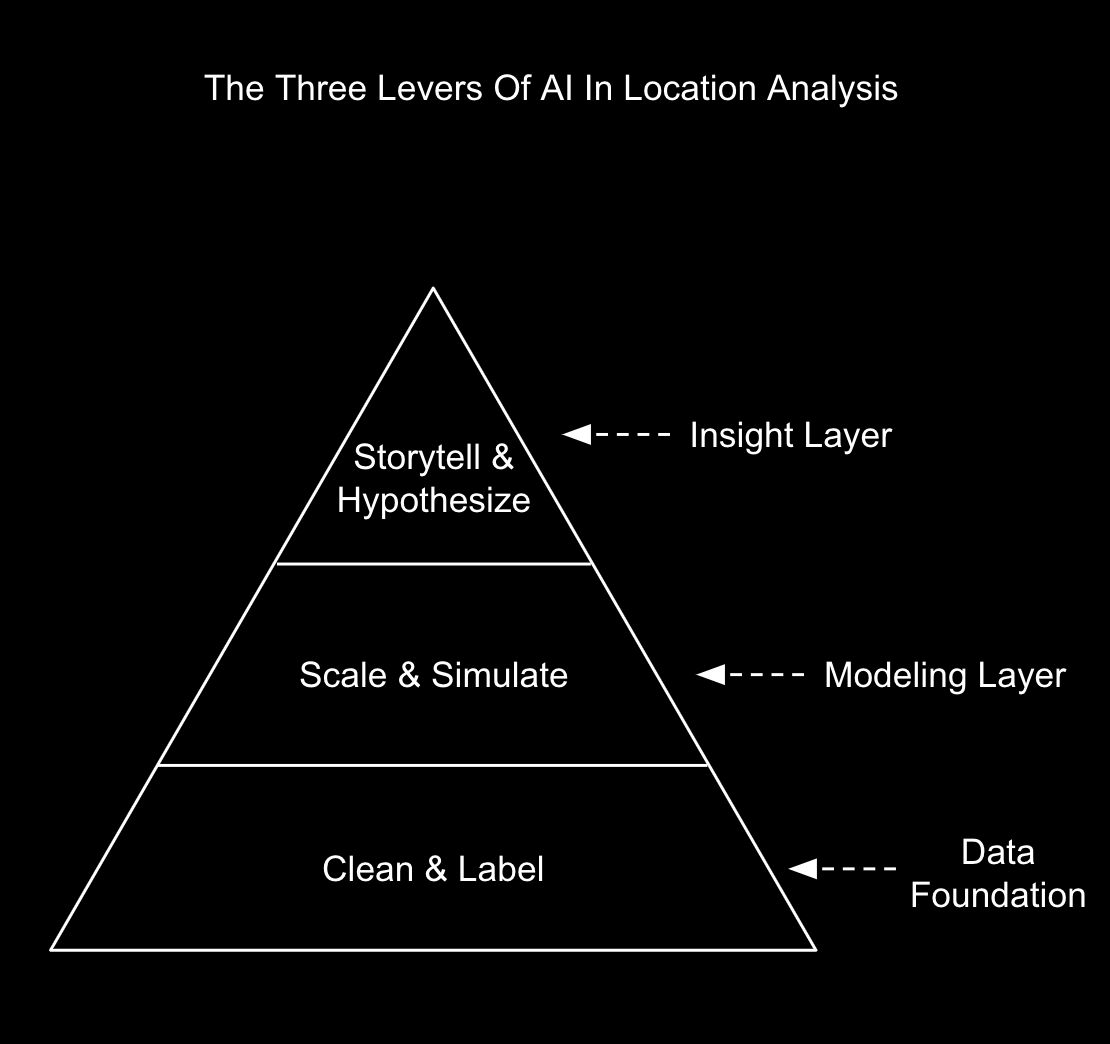
In the next few articles, we’ll explore how forward-thinking location research teams are combining data and AI to level up their site selection strategies.
{{site-selection-webinar}}
What you should do now
Whenever you're ready, here are 3 ways Spatial.ai can help:
- See PersonaLive In Action. If you'd like to segment and target your best customers using real-time behavioral data, schedule a free 30-min demo to get started.
- Subscribe To Consumer Code. If you've found this helpful, check out our newsletter and podcast where we share more consumer research and insights for retail marketers.
- Share This Post. If you know another marketer who’d enjoy reading this post, share it with them on Linkedin, X, or Facebook.
Get retail marketing tips
We email every monday with smart growth strategy ideas. Almost no promotion. Just value.
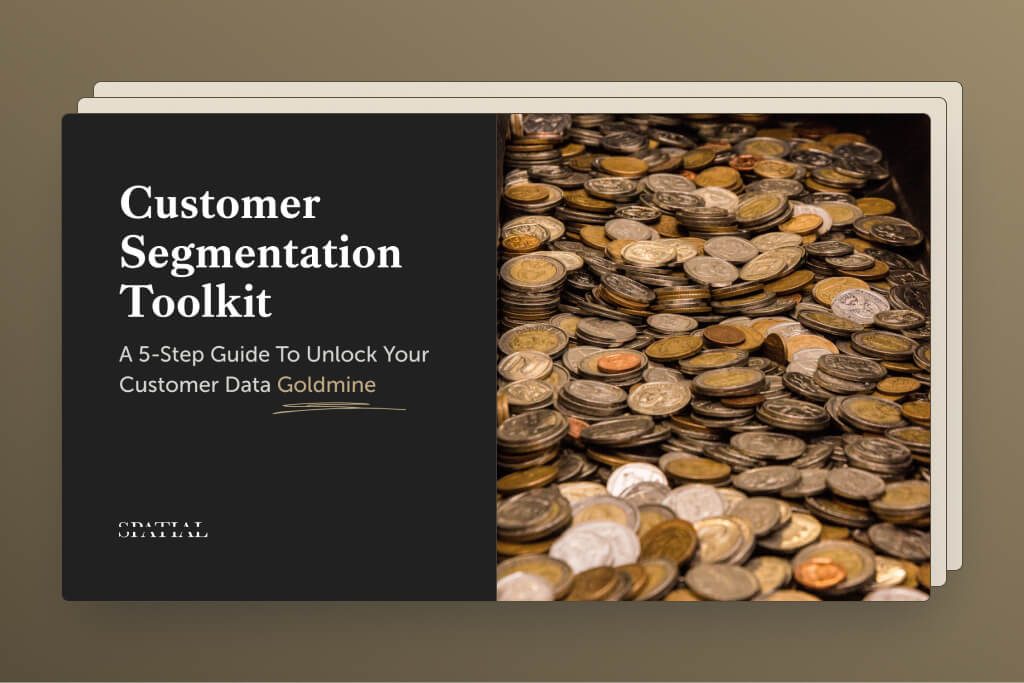
.avif)
%20(1).jpg)
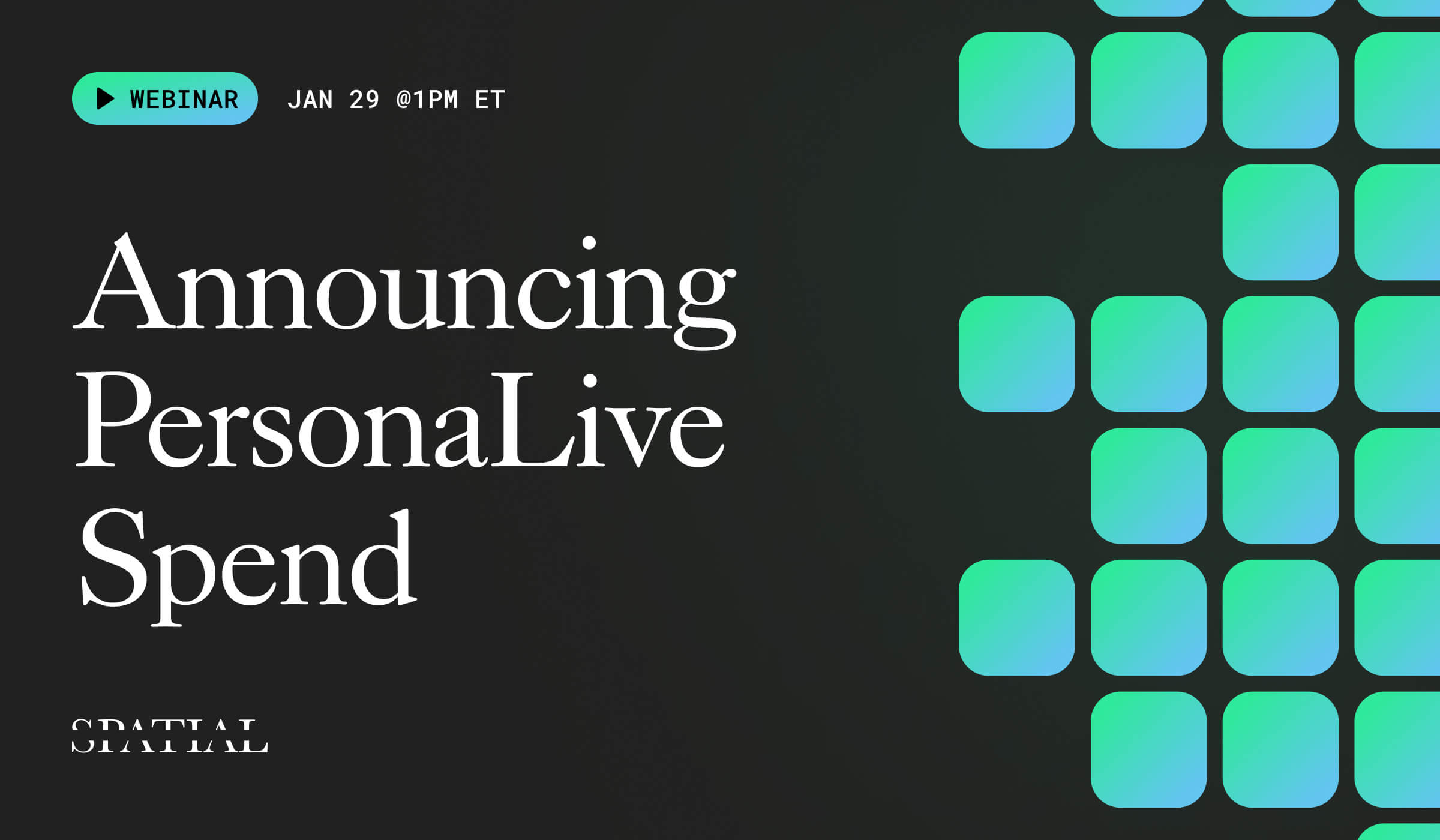
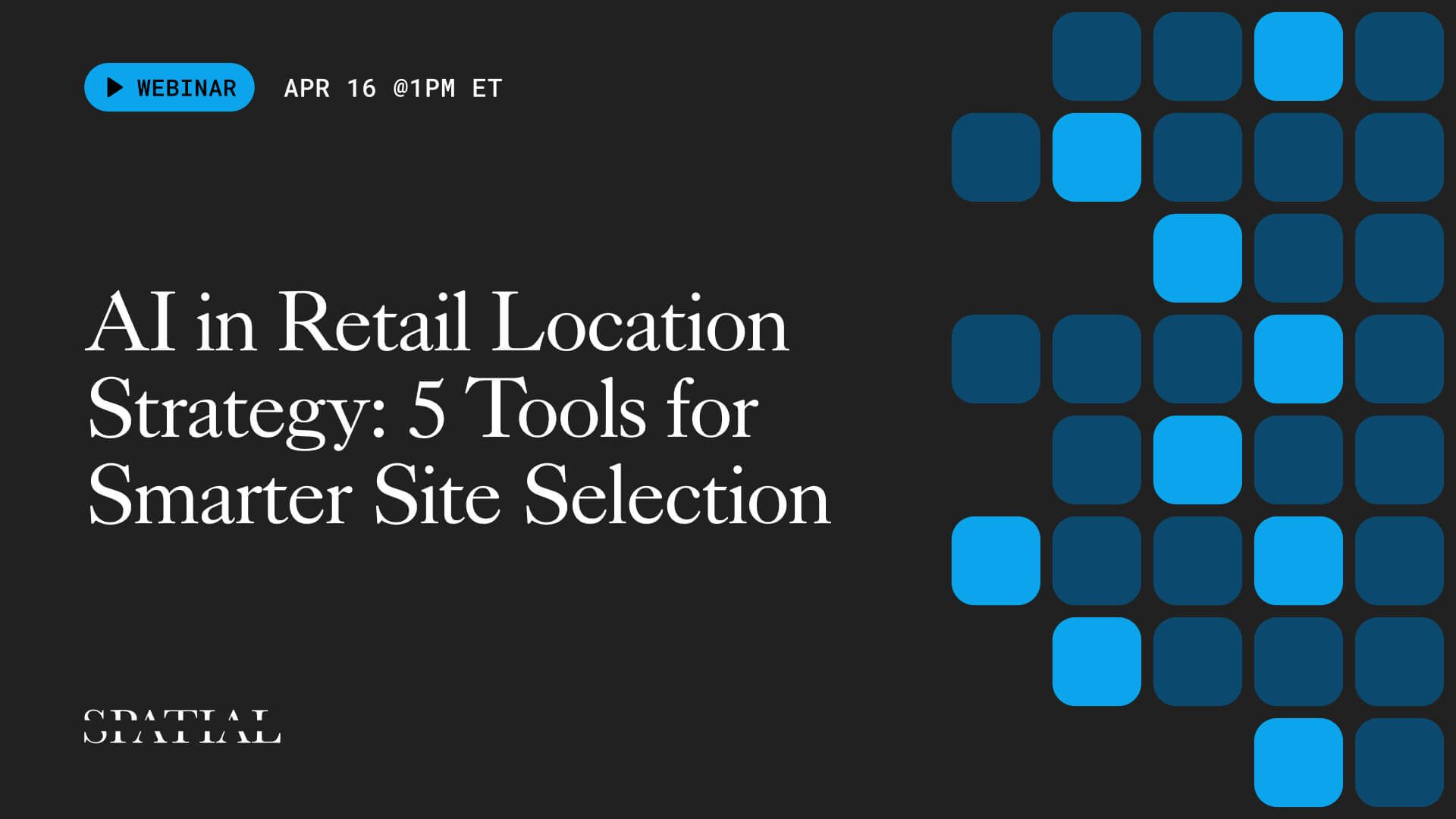

Looking to integrate AI into your location strategy? Join us for our free masterclass—AI in Retail Location Strategy: 5 Tools for Smarter Site Selection—on April 16. Register here.